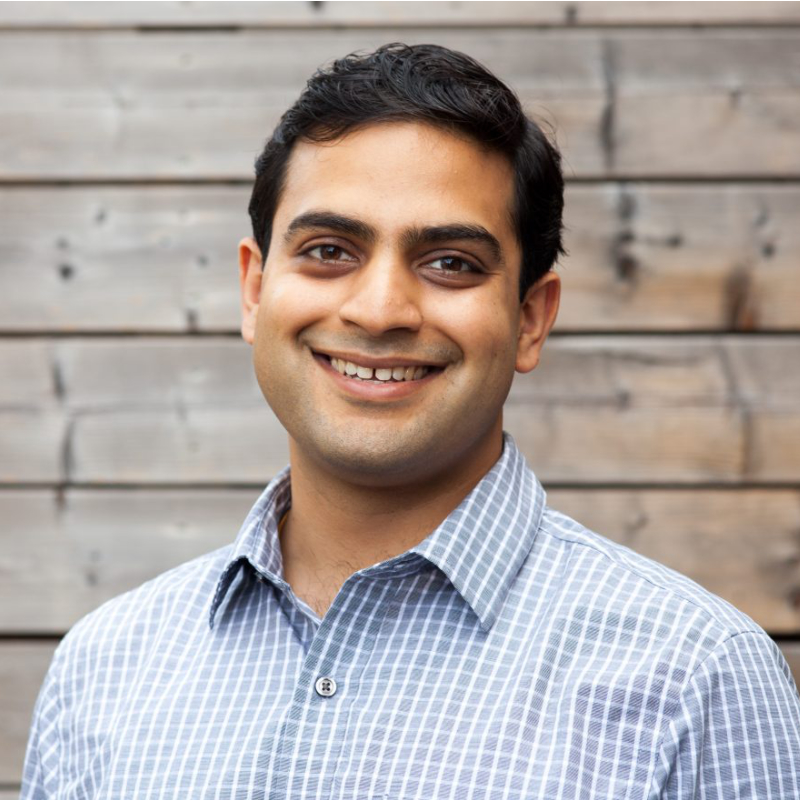
Algorithms with heart: Using machine learning to improve health care and patient outcomes
Healthcare innovation often begins at the crossroads of curiosity and necessity. For Dr. Amol Verma, a General Internal Medicine Physician at St. Michael’s Hospital, it started with a simple question: How can we deliver better care to our patients?
In the mid-2010s, Dr. Verma and his colleagues sought to measure the quality of care being provided in their hospital’s medical wards. Faced with the barrier of the lack of digitized and centralized patient records—a common challenge shared across Ontario—they were initially advised to manually review patient records.
But Dr. Verma envisioned a more efficient and impactful solution that would enable other researchers and clinicians to conduct future wide scale health care studies too. With his colleague, Dr. Fahad Razak, he co-founded GEMINI, a groundbreaking hospital data-sharing network. Concurrently, with the serendipitous rise of machine learning as a discipline, this sparked his journey into integrating health care data set analysis with machine learning.
“My entry into machine learning was pretty backwards,” Dr. Verma shares. “It started with a very applied clinical question. My colleagues and I were interested in understanding the quality of care that we were delivering on the medical wards in our hospital.”
Closing the gap in care with machine learning and clinical expertise
As a 2020 AMS Fellow in Compassionate and Artificial Intelligence (AI), a Clinician, and a Health Services Researcher, he asked a critical question. How can machine learning help predict and improve the detection of patient deterioration in hospital settings?
One major obstacle he identified was the vast variation in care patients receive, depending on which doctor is on call. Hospital stays, tests ordered, and treatments often vary due to individual clinicians’ risk tolerance and practice habits.
“This variation is what we call ‘unwarranted’ in healthcare literature. It’s not contributing to better care. And it leads to some degree of inequity in the way people receive care,” he explains.
Machine learning offers a potential solution. By processing and standardizing massive hospital datasets and analyzing key indicators of patient deterioration, machine learning models can predict when patients are at risk of worsening or require intensive care.
Through his AMS-funded research, Dr. Amol Verma and the Data Science and Advanced Analytics Team at Unity Health Toronto developed an innovative early warning system called ‘CHARTwatch’. The impact has been remarkable with a 26% reduction in unexpected deaths, achieved by combining machine learning predictions with the expertise of human clinicians. This unique synergy not only enhances accuracy, but also empowers clinicians to address unwarranted variations in care and proactively predict patient outcomes with more accuracy.
Simplifying algorithms to strengthen human connection
At the core of Dr. Verma’s work lies a commitment to compassionate care. He views machine learning as a tool rather than a solution for health care.
“Predictive analytics can allow clinicians to direct more attention to patients to create space for more human, compassionate connections,” Dr. Verma says.
Yet, scaling these solutions to clinical settings remains a challenge. Dr. Verma is tackling this simplifying machine learning tools with negligible trade-off with accuracy. For instance, a machine learning algorithm can accurately predict delirium with 100 factors. If those factors were reduced to 15 factors, there might only be a loss of 2-3% in predictive accuracy, and it becomes more practical to use. In addition, collaborating with end users, such as clinicians, can ensure that the machine learning tools are designed to be accessible and intuitive.
Dr. Verma noted that the AMS Fellowship shaped his approach to machine learning to include compassion as a key component of his research. Being an AMS Fellow provided him with the mentorship and structure for him to grow as a researcher at the start of his medical career. He also appreciates that he can turn to the AMS Healthcare community of health care leaders and researchers for collaborations and discussions.
Currently, Dr. Verma continues to co-lead GEMINI, focusing on improving how hospital data are shared to support research and drive quality improvement. He is also contributing to the implementation of AI tools to predict and prevent delirium, a leading cause of preventable harm in hospitals. Following the development of CHARTwatch, the tool has been acquired by Signal1, a company bringing predictive analytics solutions to market across North America.
Dr. Amol Verma is a General Internal Medicine Physician at St. Michael’s Hospital and a Health Services Researcher at the Li Ka Shing Knowledge Institute in Toronto. He is the Temerty Professor of AI Research and Education in Medicine at the University of Toronto and is affiliated with the Vector Institute, Ontario Health, and Digital Research Alliance of Canada. In 2015, he co-founded GEMINI, a hospital data sharing network that includes 30 hospitals across Ontario and represents about 60% of the province’s in-patient beds.
Read Dr. Amol Verma’s Publications:
Verma AA, Murray J, Greiner R, Cohen JP, Shojania KG, Ghassemi M, Straus SE, Pou-Prom C, Mamdani M. Implementing machine learning in medicine. CMAJ. 2021 Aug 30;193(34):E1351-E1357. doi: 10.1503/cmaj.202434. Epub 2021 Aug 29.
Verma AA, Stukel TA, Colacci M, Bell S, Ailon J, Friedrich JO, Murray J, Kuzulugil S, Yang Z, Lee Y, Pou-Prom C, Mamdani M. Clinical evaluation of a machine learning–based early warning system for patient deterioration. CMAJ. 2024 Sep;196(30):E1027-E1037. doi: 10.1503/cmaj.240132.
Verma AA, Pou-Prom C, McCoy LG, Murray J, Nestor B, Bell S, Mourad O, Fralick M, Friedrich J, Ghassemi M, Mamdani M. Developing and Validating a Prediction Model For Death or Critical Illness in Hospitalized Adults, an Opportunity for Human-Computer Collaboration. Crit Care Explor. 2023 May 1;5(5):e0897. doi: 10.1097/CCE.0000000000000897.
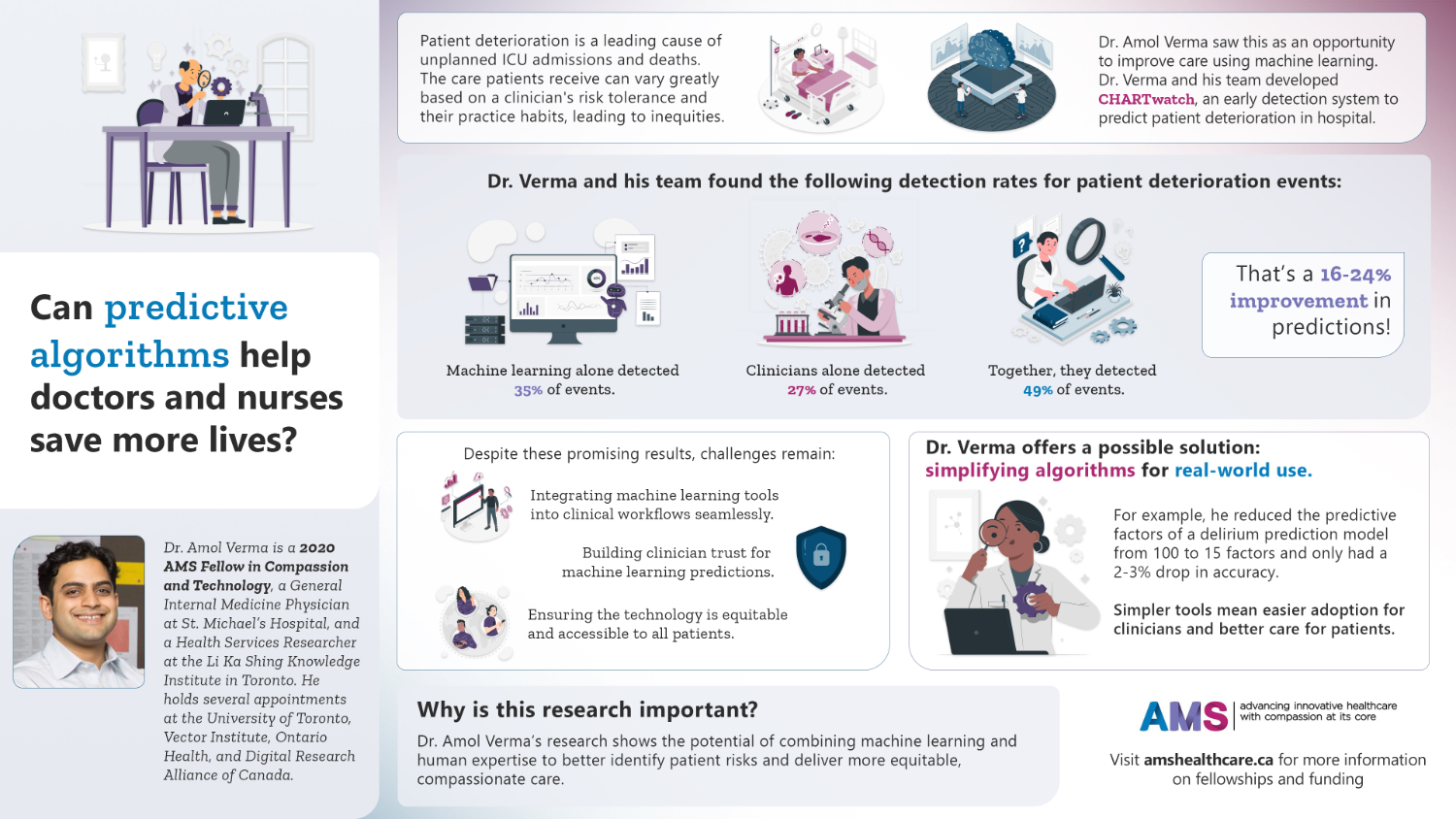